Tous les programmes traitent les données sous une forme ou une autre, et beaucoup doivent pouvoir enregistrer et récupérer ces données d'une invocation à l'autre. Python, SQLite et SQLAlchemy donnent à vos programmes une fonctionnalité de base de données, vous permettant de stocker des données dans un seul fichier sans avoir besoin d'un serveur de base de données.
Vous pouvez obtenir des résultats similaires en utilisant des fichiers plats dans n'importe quel nombre de formats, y compris CSV, JSON, XML et même des formats personnalisés. Les fichiers plats sont souvent des fichiers texte lisibles par l'homme, bien qu'ils puissent également être des données binaires, avec une structure qui peut être analysée par un programme informatique. Ci-dessous, vous explorerez l'utilisation de bases de données SQL et de fichiers plats pour le stockage et la manipulation de données et apprendrez comment décider quelle approche convient le mieux à votre programme.
Dans ce didacticiel, vous apprendrez à utiliser :
- Fichiers plats pour le stockage des données
- SQL pour améliorer l'accès aux données persistantes
- SQLite pour le stockage des données
- SQLAlchemy pour travailler avec des données en tant qu'objets Python
Vous pouvez obtenir tout le code et les données que vous verrez dans ce didacticiel en cliquant sur le lien ci-dessous :
Téléchargez l'exemple de code : Cliquez ici pour obtenir le code que vous utiliserez pour en savoir plus sur la gestion des données avec SQLite et SQLAlchemy dans ce didacticiel.
Utilisation de fichiers plats pour le stockage de données
Un fichier plat est un fichier contenant des données sans hiérarchie interne et généralement sans références à des fichiers externes. Les fichiers plats contiennent des caractères lisibles par l'homme et sont très utiles pour créer et lire des données. Parce qu'ils n'ont pas besoin d'utiliser des largeurs de champ fixes, les fichiers plats utilisent souvent d'autres structures pour permettre à un programme d'analyser le texte.
Par exemple, les fichiers de valeurs séparées par des virgules (CSV) sont des lignes de texte brut dans lesquelles le caractère virgule sépare les éléments de données. Chaque ligne de texte représente une ligne de données et chaque valeur séparée par des virgules est un champ dans cette ligne. Le séparateur de caractères virgule indique la limite entre les valeurs de données.
Python excelle dans la lecture et l'enregistrement de fichiers. Être capable de lire des fichiers de données avec Python vous permet de restaurer une application dans un état utile lorsque vous la réexécutez ultérieurement. La possibilité d'enregistrer des données dans un fichier vous permet de partager les informations du programme entre les utilisateurs et les sites sur lesquels l'application s'exécute.
Avant qu'un programme puisse lire un fichier de données, il doit être capable de comprendre les données. Habituellement, cela signifie que le fichier de données doit avoir une structure que l'application peut utiliser pour lire et analyser le texte dans le fichier.
Ci-dessous se trouve un fichier CSV nommé author_book_publisher.csv
, utilisé par le premier exemple de programme de ce tutoriel :
first_name,last_name,title,publisher
Isaac,Asimov,Foundation,Random House
Pearl,Buck,The Good Earth,Random House
Pearl,Buck,The Good Earth,Simon & Schuster
Tom,Clancy,The Hunt For Red October,Berkley
Tom,Clancy,Patriot Games,Simon & Schuster
Stephen,King,It,Random House
Stephen,King,It,Penguin Random House
Stephen,King,Dead Zone,Random House
Stephen,King,The Shining,Penguin Random House
John,Le Carre,"Tinker, Tailor, Soldier, Spy: A George Smiley Novel",Berkley
Alex,Michaelides,The Silent Patient,Simon & Schuster
Carol,Shaben,Into The Abyss,Simon & Schuster
La première ligne fournit une liste de champs séparés par des virgules, qui sont les noms de colonne pour les données qui suivent dans les lignes restantes. Le reste des lignes contient les données, chaque ligne représentant un seul enregistrement.
Remarque : Bien que les auteurs, les livres et les éditeurs soient tous réels, les relations entre les livres et les éditeurs sont fictives et ont été créées pour les besoins de ce didacticiel.
Ensuite, vous examinerez certains des avantages et des inconvénients de l'utilisation de fichiers plats comme le CSV ci-dessus pour travailler avec vos données.
Avantages des fichiers plats
Travailler avec des données dans des fichiers plats est gérable et simple à mettre en œuvre. Avoir les données dans un format lisible par l'homme est utile non seulement pour créer le fichier de données avec un éditeur de texte, mais aussi pour examiner les données et rechercher d'éventuelles incohérences ou problèmes.
De nombreuses applications peuvent exporter des versions de fichier plat des données générées par le fichier. Par exemple, Excel peut importer ou exporter un fichier CSV vers et depuis une feuille de calcul. Les fichiers plats ont également l'avantage d'être autonomes et transférables si vous souhaitez partager les données.
Presque tous les langages de programmation disposent d'outils et de bibliothèques qui facilitent le travail avec les fichiers CSV. Python a le csv
intégré module et le puissant module pandas disponibles, faisant du travail avec des fichiers CSV une solution puissante.
Inconvénients des fichiers plats
Les avantages de travailler avec des fichiers plats commencent à diminuer à mesure que les données deviennent plus volumineuses. Les fichiers volumineux sont toujours lisibles par l'homme, mais les modifier pour créer des données ou rechercher des problèmes devient une tâche plus difficile. Si votre application modifie les données du fichier, une solution consiste à lire l'intégralité du fichier en mémoire, à apporter les modifications et à écrire les données dans un autre fichier.
Un autre problème lié à l'utilisation de fichiers plats est que vous devrez créer et maintenir explicitement toutes les relations entre des parties de vos données et le programme d'application dans la syntaxe du fichier. De plus, vous devrez générer du code dans votre application pour utiliser ces relations.
Une dernière complication est que les personnes avec lesquelles vous souhaitez partager votre fichier de données devront également connaître et agir sur les structures et les relations que vous avez créées dans les données. Pour accéder aux informations, ces utilisateurs devront comprendre non seulement la structure des données, mais également les outils de programmation nécessaires pour y accéder.
Exemple de fichier plat
Le programme exemple examples/example_1/main.py
utilise le author_book_publisher.csv
fichier pour obtenir les données et les relations qu'il contient. Ce fichier CSV contient une liste des auteurs, des livres qu'ils ont publiés et des éditeurs pour chacun des livres.
Remarque : Les fichiers de données utilisés dans les exemples sont disponibles dans le dossier project/data
annuaire. Il y a aussi un fichier programme dans le project/build_data
répertoire qui génère les données. Cette application est utile si vous modifiez les données et souhaitez revenir à un état connu.
Pour accéder aux fichiers de données utilisés dans cette section et tout au long du didacticiel, cliquez sur le lien ci-dessous :
Téléchargez l'exemple de code : Cliquez ici pour obtenir le code que vous utiliserez pour en savoir plus sur la gestion des données avec SQLite et SQLAlchemy dans ce didacticiel.
Le fichier CSV présenté ci-dessus est un assez petit fichier de données contenant seulement quelques auteurs, livres et éditeurs. Vous devriez également remarquer certaines choses concernant les données :
-
Les auteurs Stephen King et Tom Clancy apparaissent plus d'une fois car plusieurs livres qu'ils ont publiés sont représentés dans les données.
-
Les auteurs Stephen King et Pearl Buck ont le même livre publié par plus d'un éditeur.
Ces champs de données dupliqués créent des relations entre d'autres parties des données. Un auteur peut écrire plusieurs livres et un éditeur peut travailler avec plusieurs auteurs. Les auteurs et les éditeurs partagent des relations avec des livres individuels.
Les relations dans le author_book_publisher.csv
sont représentés par des champs qui apparaissent plusieurs fois dans différentes lignes du fichier de données. En raison de cette redondance des données, les données représentent plus d'un seul tableau à deux dimensions. Vous en verrez plus lorsque vous utiliserez le fichier pour créer un fichier de base de données SQLite.
Le programme exemple examples/example_1/main.py
utilise les relations intégrées dans le author_book_publisher.csv
fichier pour générer des données. Il présente d'abord une liste des auteurs et le nombre de livres que chacun a écrits. Il affiche ensuite une liste d'éditeurs et le nombre d'auteurs pour lesquels chacun a publié des livres.
Il utilise également le treelib
module pour afficher une arborescence des auteurs, des livres et des éditeurs.
Enfin, il ajoute un nouveau livre aux données et réaffiche l'arborescence avec le nouveau livre en place. Voici le main()
fonction de point d'entrée pour ce programme :
1def main():
2 """The main entry point of the program"""
3 # Get the resources for the program
4 with resources.path(
5 "project.data", "author_book_publisher.csv"
6 ) as filepath:
7 data = get_data(filepath)
8
9 # Get the number of books printed by each publisher
10 books_by_publisher = get_books_by_publisher(data, ascending=False)
11 for publisher, total_books in books_by_publisher.items():
12 print(f"Publisher: {publisher}, total books: {total_books}")
13 print()
14
15 # Get the number of authors each publisher publishes
16 authors_by_publisher = get_authors_by_publisher(data, ascending=False)
17 for publisher, total_authors in authors_by_publisher.items():
18 print(f"Publisher: {publisher}, total authors: {total_authors}")
19 print()
20
21 # Output hierarchical authors data
22 output_author_hierarchy(data)
23
24 # Add a new book to the data structure
25 data = add_new_book(
26 data,
27 author_name="Stephen King",
28 book_title="The Stand",
29 publisher_name="Random House",
30 )
31
32 # Output the updated hierarchical authors data
33 output_author_hierarchy(data)
Le code Python ci-dessus suit les étapes suivantes :
- Lignes 4 à 7 lire le
author_book_publisher.csv
fichier dans un pandas DataFrame. - Lignes 10 à 13 imprimer le nombre de livres publiés par chaque éditeur.
- Lignes 16 à 19 imprimer le nombre d'auteurs associés à chaque éditeur.
- Ligne 22 affiche les données du livre sous la forme d'une hiérarchie triée par auteurs.
- Lignes 25 à 30 ajouter un nouveau livre à la structure en mémoire.
- Ligne 33 affiche les données du livre sous la forme d'une hiérarchie triée par auteurs, y compris le livre nouvellement ajouté.
L'exécution de ce programme génère la sortie suivante :
$ python main.py
Publisher: Simon & Schuster, total books: 4
Publisher: Random House, total books: 4
Publisher: Penguin Random House, total books: 2
Publisher: Berkley, total books: 2
Publisher: Simon & Schuster, total authors: 4
Publisher: Random House, total authors: 3
Publisher: Berkley, total authors: 2
Publisher: Penguin Random House, total authors: 1
Authors
├── Alex Michaelides
│ └── The Silent Patient
│ └── Simon & Schuster
├── Carol Shaben
│ └── Into The Abyss
│ └── Simon & Schuster
├── Isaac Asimov
│ └── Foundation
│ └── Random House
├── John Le Carre
│ └── Tinker, Tailor, Soldier, Spy: A George Smiley Novel
│ └── Berkley
├── Pearl Buck
│ └── The Good Earth
│ ├── Random House
│ └── Simon & Schuster
├── Stephen King
│ ├── Dead Zone
│ │ └── Random House
│ ├── It
│ │ ├── Penguin Random House
│ │ └── Random House
│ └── The Shining
│ └── Penguin Random House
└── Tom Clancy
├── Patriot Games
│ └── Simon & Schuster
└── The Hunt For Red October
└── Berkley
La hiérarchie des auteurs ci-dessus est présentée deux fois dans la sortie, avec l'ajout de The Stand de Stephen King , publié par Random House. La sortie réelle ci-dessus a été modifiée et affiche uniquement la première sortie de la hiérarchie pour économiser de l'espace.
main()
appelle d'autres fonctions pour effectuer le gros du travail. La première fonction qu'il appelle est get_data()
:
def get_data(filepath):
"""Get book data from the csv file"""
return pd.read_csv(filepath)
Cette fonction prend le chemin d'accès au fichier CSV et utilise pandas pour le lire dans un pandas DataFrame, qu'il retransmet ensuite à l'appelant. La valeur de retour de cette fonction devient la structure de données passée aux autres fonctions qui composent le programme.
get_books_by_publisher()
calcule le nombre de livres publiés par chaque éditeur. La série pandas résultante utilise la fonctionnalité pandas GroupBy pour regrouper par éditeur, puis trier en fonction du ascending
drapeau :
def get_books_by_publisher(data, ascending=True):
"""Return the number of books by each publisher as a pandas series"""
return data.groupby("publisher").size().sort_values(ascending=ascending)
get_authors_by_publisher()
fait essentiellement la même chose que la fonction précédente, mais pour les auteurs :
def get_authors_by_publisher(data, ascending=True):
"""Returns the number of authors by each publisher as a pandas series"""
return (
data.assign(name=data.first_name.str.cat(data.last_name, sep=" "))
.groupby("publisher")
.nunique()
.loc[:, "name"]
.sort_values(ascending=ascending)
)
add_new_book()
crée un nouveau livre dans le pandas DataFrame. Le code vérifie si l'auteur, le livre ou l'éditeur existe déjà. Si ce n'est pas le cas, il crée un nouveau livre et l'ajoute au pandas DataFrame :
def add_new_book(data, author_name, book_title, publisher_name):
"""Adds a new book to the system"""
# Does the book exist?
first_name, _, last_name = author_name.partition(" ")
if any(
(data.first_name == first_name)
& (data.last_name == last_name)
& (data.title == book_title)
& (data.publisher == publisher_name)
):
return data
# Add the new book
return data.append(
{
"first_name": first_name,
"last_name": last_name,
"title": book_title,
"publisher": publisher_name,
},
ignore_index=True,
)
output_author_hierarchy()
utilise for
imbriqué boucles pour parcourir les niveaux de la structure de données. Il utilise alors le treelib
module pour générer une liste hiérarchique des auteurs, des livres qu'ils ont publiés et des éditeurs qui ont publié ces livres :
def output_author_hierarchy(data):
"""Output the data as a hierarchy list of authors"""
authors = data.assign(
name=data.first_name.str.cat(data.last_name, sep=" ")
)
authors_tree = Tree()
authors_tree.create_node("Authors", "authors")
for author, books in authors.groupby("name"):
authors_tree.create_node(author, author, parent="authors")
for book, publishers in books.groupby("title")["publisher"]:
book_id = f"{author}:{book}"
authors_tree.create_node(book, book_id, parent=author)
for publisher in publishers:
authors_tree.create_node(publisher, parent=book_id)
# Output the hierarchical authors data
authors_tree.show()
Cette application fonctionne bien et illustre la puissance dont vous disposez avec le module pandas. Le module fournit d'excellentes fonctionnalités pour lire un fichier CSV et interagir avec les données.
Continuons et créons un programme fonctionnant de manière identique en utilisant Python, une version de base de données SQLite des données d'auteur et de publication, et SQLAlchemy pour interagir avec ces données.
Utiliser SQLite pour conserver les données
Comme vous l'avez vu précédemment, il y a des données redondantes dans le author_book_publisher.csv
dossier. Par exemple, toutes les informations sur The Good Earth de Pearl Buck est répertorié deux fois car deux éditeurs différents ont publié le livre.
Imaginez si ce fichier de données contenait davantage de données connexes, telles que l'adresse et le numéro de téléphone de l'auteur, les dates de publication et les ISBN des livres, ou les adresses, les numéros de téléphone et peut-être les revenus annuels des éditeurs. Ces données seraient dupliquées pour chaque élément de données racine, comme l'auteur, le livre ou l'éditeur.
Il est possible de créer des données de cette façon, mais ce serait exceptionnellement difficile à manier. Réfléchissez aux problèmes de mise à jour de ce fichier de données. Et si Stephen King voulait changer de nom ? Vous devrez mettre à jour plusieurs enregistrements contenant son nom et vous assurer qu'il n'y a pas de fautes de frappe.
Pire que la duplication des données serait la complexité d'ajouter d'autres relations aux données. Et si vous décidiez d'ajouter des numéros de téléphone pour les auteurs, et qu'ils avaient des numéros de téléphone pour la maison, le travail, le mobile et peut-être plus ? Chaque nouvelle relation que vous voudriez ajouter pour un élément racine multiplierait le nombre d'enregistrements par le nombre d'éléments dans cette nouvelle relation.
Ce problème est l'une des raisons pour lesquelles des relations existent dans les systèmes de base de données. Un sujet important dans l'ingénierie des bases de données est la normalisation des bases de données , ou le processus de séparation des données pour réduire la redondance et augmenter l'intégrité. Lorsqu'une structure de base de données est étendue avec de nouveaux types de données, sa normalisation préalable réduit au minimum les modifications apportées à la structure existante.
La base de données SQLite est disponible en Python et, selon la page d'accueil de SQLite, elle est plus utilisée que tous les autres systèmes de base de données combinés. Il offre un système de gestion de base de données relationnelle (RDBMS) complet qui fonctionne avec un seul fichier pour maintenir toutes les fonctionnalités de la base de données.
Il présente également l'avantage de ne pas nécessiter de serveur de base de données séparé pour fonctionner. Le format de fichier de base de données est multiplateforme et accessible à tout langage de programmation prenant en charge SQLite.
Toutes ces informations sont intéressantes, mais en quoi sont-elles pertinentes pour l'utilisation de fichiers plats pour le stockage de données ? Vous le découvrirez ci-dessous !
Création d'une structure de base de données
L'approche par force brute pour obtenir le author_book_publisher.csv
données dans une base de données SQLite serait de créer une seule table correspondant à la structure du fichier CSV. Faire cela ignorerait une bonne partie de la puissance de SQLite.
Bases de données relationnelles fournir un moyen de stocker des données structurées dans des tables et d'établir des relations entre ces tables. Ils utilisent généralement le langage SQL (Structured Query Language) comme principal moyen d'interagir avec les données. Il s'agit d'une simplification excessive de ce que fournissent les SGBDR, mais c'est suffisant pour les besoins de ce didacticiel.
Une base de données SQLite prend en charge l'interaction avec la table de données à l'aide de SQL. Non seulement un fichier de base de données SQLite contient les données, mais il a également une manière standardisée d'interagir avec les données. Cette prise en charge est intégrée dans le fichier, ce qui signifie que tout langage de programmation pouvant utiliser un fichier SQLite peut également utiliser SQL pour travailler avec.
Interagir avec une base de données avec SQL
SQL est un langage déclaratif utilisé pour créer, gérer et interroger les données contenues dans une base de données. Un langage déclaratif décrit quoi doit être accompli plutôt que comment cela devrait être accompli. Vous verrez des exemples d'instructions SQL plus tard lorsque vous arriverez à créer des tables de base de données.
Structurer une base de données avec SQL
Pour tirer parti de la puissance de SQL, vous devrez appliquer une certaine normalisation de la base de données aux données du author_book_publisher.csv
dossier. Pour ce faire, vous allez séparer les auteurs, les livres et les éditeurs dans des tables de base de données distinctes.
Conceptuellement, les données sont stockées dans la base de données dans des structures de table à deux dimensions. Chaque table se compose de rangées d'enregistrements , et chaque enregistrement se compose de colonnes ou de champs , contenant des données.
Les données contenues dans les champs sont de types prédéfinis, notamment du texte, des entiers, des flottants, etc. Les fichiers CSV sont différents car tous les champs sont du texte et doivent être analysés par un programme pour qu'un type de données leur soit attribué.
Chaque enregistrement de la table a une clé primaire défini pour donner à un enregistrement un identifiant unique. La clé primaire est similaire à la clé d'un dictionnaire Python. Le moteur de base de données lui-même génère souvent la clé primaire sous la forme d'une valeur entière incrémentielle pour chaque enregistrement inséré dans la table de la base de données.
Bien que la clé primaire soit souvent générée automatiquement par le moteur de base de données, ce n'est pas obligatoire. Si les données stockées dans un champ sont uniques parmi toutes les autres données de la table dans ce champ, il peut s'agir de la clé primaire. Par exemple, une table contenant des données sur les livres pourrait utiliser l'ISBN du livre comme clé primaire.
Créer des tableaux avec SQL
Voici comment vous pouvez créer les trois tables représentant les auteurs, les livres et les éditeurs dans le fichier CSV à l'aide d'instructions SQL :
CREATE TABLE author (
author_id INTEGER NOT NULL PRIMARY KEY,
first_name VARCHAR,
last_name VARCHAR
);
CREATE TABLE book (
book_id INTEGER NOT NULL PRIMARY KEY,
author_id INTEGER REFERENCES author,
title VARCHAR
);
CREATE TABLE publisher (
publisher_id INTEGER NOT NULL PRIMARY KEY,
name VARCHAR
);
Notez qu'il n'y a pas d'opérations sur les fichiers, pas de variables créées et pas de structures pour les contenir. Les instructions décrivent uniquement le résultat souhaité :la création d'une table avec des attributs particuliers. Le moteur de base de données détermine comment procéder.
Une fois que vous avez créé et rempli ce tableau avec les données d'auteur du author_book_publisher.csv
fichier, vous pouvez y accéder à l'aide d'instructions SQL. L'instruction suivante (également appelée requête ) utilise le caractère générique (*
) pour obtenir toutes les données dans le author
table et affichez-la :
SELECT * FROM author;
Vous pouvez utiliser le sqlite3
outil en ligne de commande pour interagir avec author_book_publisher.db
fichier de base de données dans le project/data
répertoire :
$ sqlite3 author_book_publisher.db
Une fois que l'outil de ligne de commande SQLite est en cours d'exécution avec la base de données ouverte, vous pouvez entrer des commandes SQL. Voici la commande SQL ci-dessus et sa sortie, suivie du .q
commande pour quitter le programme :
sqlite> SELECT * FROM author;
1|Isaac|Asimov
2|Pearl|Buck
3|Tom|Clancy
4|Stephen|King
5|John|Le Carre
6|Alex|Michaelides
7|Carol|Shaben
sqlite> .q
Notez que chaque auteur n'existe qu'une seule fois dans la table. Contrairement au fichier CSV, qui comportait plusieurs entrées pour certains auteurs, ici, un seul enregistrement unique par auteur est nécessaire.
Maintenir une base de données avec SQL
SQL fournit des moyens de travailler avec des bases de données et des tables existantes en insérant de nouvelles données et en mettant à jour ou en supprimant des données existantes. Voici un exemple d'instruction SQL pour insérer un nouvel auteur dans le author
tableau :
INSERT INTO author
(first_name, last_name)
VALUES ('Paul', 'Mendez');
Cette instruction SQL insère les valeurs 'Paul
‘ et ‘Mendez
‘ dans les colonnes respectives first_name
et last_name
de l'author
table.
Notez que le author_id
la colonne n'est pas spécifiée. Étant donné que cette colonne est la clé primaire, le moteur de base de données génère la valeur et l'insère dans le cadre de l'exécution de l'instruction.
La mise à jour des enregistrements dans une table de base de données est un processus simple. Par exemple, supposons que Stephen King veuille être connu sous son pseudonyme, Richard Bachman. Voici une instruction SQL pour mettre à jour l'enregistrement de la base de données :
UPDATE author
SET first_name = 'Richard', last_name = 'Bachman'
WHERE first_name = 'Stephen' AND last_name = 'King';
L'instruction SQL localise l'enregistrement unique pour 'Stephen King'
en utilisant l'instruction conditionnelle WHERE first_name = 'Stephen' AND last_name = 'King'
puis met à jour le first_name
et last_name
champs avec les nouvelles valeurs. SQL utilise le signe égal (=
) en tant qu'opérateur de comparaison et opérateur d'affectation.
Vous pouvez également supprimer des enregistrements d'une base de données. Voici un exemple d'instruction SQL pour supprimer un enregistrement de l'author
tableau :
DELETE FROM author
WHERE first_name = 'Paul'
AND last_name = 'Mendez';
Cette instruction SQL supprime une seule ligne de author
table où le first_name
est égal à 'Paul'
et le last_name
est égal à 'Mendez'
.
Soyez prudent lorsque vous supprimez des enregistrements ! Les conditions que vous définissez doivent être aussi précises que possible. Une condition trop large peut entraîner la suppression de plus d'enregistrements que prévu. Par exemple, si la condition était basée uniquement sur la ligne first_name = 'Paul'
, alors tous les auteurs dont le prénom est Paul seraient supprimés de la base de données.
Remarque : Pour éviter la suppression accidentelle d'enregistrements, de nombreuses applications n'autorisent pas du tout les suppressions. Au lieu de cela, l'enregistrement a une autre colonne pour indiquer s'il est utilisé ou non. Cette colonne peut être nommée active
et contiennent une valeur qui prend la valeur True ou False, indiquant si l'enregistrement doit être inclus lors de l'interrogation de la base de données.
Par exemple, la requête SQL ci-dessous obtiendrait toutes les colonnes pour tous les enregistrements actifs dans some_table
:
SELECT
*
FROM some_table
WHERE active = 1;
SQLite n'a pas de type de données booléen, donc le active
colonne est représentée par un entier avec une valeur de 0
ou 1
pour indiquer l'état de l'enregistrement. D'autres systèmes de base de données peuvent ou non avoir des types de données booléens natifs.
Il est tout à fait possible de créer des applications de base de données en Python en utilisant des instructions SQL directement dans le code. Cela renvoie les données à l'application sous la forme d'une liste de listes ou d'une liste de dictionnaires.
L'utilisation de SQL brut est une manière parfaitement acceptable de travailler avec les données renvoyées par les requêtes à la base de données. Cependant, plutôt que de faire cela, vous allez passer directement à l'utilisation de SQLAlchemy pour travailler avec des bases de données.
Établir des relations
Les relations sont une autre fonctionnalité des systèmes de bases de données que vous pourriez trouver encore plus puissante et utile que la persistance et la récupération des données. . Les bases de données qui prennent en charge les relations vous permettent de diviser les données en plusieurs tables et d'établir des connexions entre elles.
Les données dans le author_book_publisher.csv
Le fichier représente les données et les relations en dupliquant les données. Une base de données gère cela en divisant les données en trois tables :author
, book
, et publisher
—et établir des relations entre eux.
Après avoir rassemblé toutes les données souhaitées au même endroit dans le fichier CSV, pourquoi voudriez-vous les diviser en plusieurs tables ? Ne serait-ce pas plus de travail à créer et à reconstituer ? C'est vrai dans une certaine mesure, mais les avantages de décomposer les données et de les reconstituer à l'aide de SQL pourraient vous séduire !
Relations un-à-plusieurs
Un un-à-plusieurs relation est comme celle d'un client commandant des articles en ligne. Un client peut avoir plusieurs commandes, mais chaque commande appartient à un client. Le author_book_publisher.db
base de données a une relation un-à-plusieurs sous la forme d'auteurs et de livres. Chaque auteur peut écrire plusieurs livres, mais chaque livre est écrit par un seul auteur.
Comme vous l'avez vu dans la création de table ci-dessus, la mise en œuvre de ces entités distinctes consiste à placer chacune dans une table de base de données, une pour les auteurs et une pour les livres. Mais comment la relation un-à-plusieurs entre ces deux tables est-elle implémentée ?
N'oubliez pas que chaque table d'une base de données a un champ désigné comme clé primaire pour cette table. Chaque table ci-dessus a un champ de clé primaire nommé selon ce modèle :<table name>_id
.
Le book
la table ci-dessus contient un champ, author_id
, qui fait référence à l'author
table. Le author_id
champ établit une relation un-à-plusieurs entre les auteurs et les livres qui ressemble à ceci :

Le diagramme ci-dessus est un simple diagramme entité-relation (ERD) créé avec l'application JetBrains DataGrip montrant les tables author
et book
sous forme de boîtes avec leurs champs de clé primaire et de données respectifs. Deux éléments graphiques ajoutent des informations sur la relation :
-
Les petites icônes de clé jaune et bleue indiquez respectivement les clés primaire et étrangère de la table.
-
La flèche reliant
book
àauthor
indique la relation entre les tables en fonction duauthor_id
clé étrangère dans lebook
tableau.
Lorsque vous ajoutez un nouveau livre au book
table, les données incluent un author_id
valeur pour un auteur existant dans author
table. De cette façon, tous les livres écrits par un auteur ont une relation de recherche avec cet auteur unique.
Maintenant que vous avez des tableaux séparés pour les auteurs et les livres, comment utilisez-vous la relation entre eux ? SQL prend en charge ce qu'on appelle un JOIN
opération, que vous pouvez utiliser pour indiquer à la base de données comment connecter deux ou plusieurs tables.
La requête SQL ci-dessous rejoint le author
et book
table ensemble à l'aide de l'application de ligne de commande SQLite :
sqlite> SELECT
...> a.first_name || ' ' || a.last_name AS author_name,
...> b.title AS book_title
...> FROM author a
...> JOIN book b ON b.author_id = a.author_id
...> ORDER BY a.last_name ASC;
Isaac Asimov|Foundation
Pearl Buck|The Good Earth
Tom Clancy|The Hunt For Red October
Tom Clancy|Patriot Games
Stephen King|It
Stephen King|Dead Zone
Stephen King|The Shining
John Le Carre|Tinker, Tailor, Soldier, Spy: A George Smiley Novel
Alex Michaelides|The Silent Patient
Carol Shaben|Into The Abyss
La requête SQL ci-dessus rassemble les informations de la table author et book en joignant les tables à l'aide de la relation établie entre les deux. La concaténation de chaînes SQL attribue le nom complet de l'auteur à l'alias author_name
. Les données recueillies par la requête sont triées par ordre croissant par le last_name
champ.
Il y a quelques éléments à remarquer dans l'instruction SQL. Premièrement, les auteurs sont présentés par leur nom complet dans une seule colonne et triés par leur nom de famille. En outre, les auteurs apparaissent plusieurs fois dans la sortie en raison de la relation un-à-plusieurs. Le nom d'un auteur est dupliqué pour chaque livre qu'il a écrit dans la base de données.
En créant des tableaux séparés pour les auteurs et les livres et en établissant la relation entre eux, vous avez réduit la redondance dans les données. Désormais, vous n'avez plus qu'à modifier les données d'un auteur à un seul endroit, et cette modification apparaît dans toute requête SQL accédant aux données.
Relations plusieurs à plusieurs
Plusieurs à plusieurs des relations existent dans author_book_publisher.db
base de données entre auteurs et éditeurs ainsi qu'entre livres et éditeurs. Un auteur peut travailler avec plusieurs éditeurs et un éditeur peut travailler avec plusieurs auteurs. De même, un livre peut être publié par plusieurs éditeurs et un éditeur peut publier plusieurs livres.
Handling this situation in the database is more involved than a one-to-many relationship because the relationship goes both ways. Many-to-many relationships are created by an association table acting as a bridge between the two related tables.
The association table contains at least two foreign key fields, which are the primary keys of each of the two associated tables. This SQL statement creates the association table relating the author
and publisher
tables:
CREATE TABLE author_publisher (
author_id INTEGER REFERENCES author,
publisher_id INTEGER REFERENCES publisher
);
The SQL statements create a new author_publisher
table referencing the primary keys of the existing author
and publisher
les tables. The author_publisher
table is an association table establishing relationships between an author and a publisher.
Because the relationship is between two primary keys, there’s no need to create a primary key for the association table itself. The combination of the two related keys creates a unique identifier for a row of data.
As before, you use the JOIN
keyword to connect two tables together. Connecting the author
table to the publisher
table is a two-step process:
JOIN
theauthor
table with theauthor_publisher
table.JOIN
theauthor_publisher
table with thepublisher
table.
The author_publisher
association table provides the bridge through which the JOIN
connects the two tables. Here’s an example SQL query returning a list of authors and the publishers publishing their books:
1sqlite> SELECT
2 ...> a.first_name || ' ' || a.last_name AS author_name,
3 ...> p.name AS publisher_name
4 ...> FROM author a
5 ...> JOIN author_publisher ap ON ap.author_id = a.author_id
6 ...> JOIN publisher p ON p.publisher_id = ap.publisher_id
7 ...> ORDER BY a.last_name ASC;
8Isaac Asimov|Random House
9Pearl Buck|Random House
10Pearl Buck|Simon & Schuster
11Tom Clancy|Berkley
12Tom Clancy|Simon & Schuster
13Stephen King|Random House
14Stephen King|Penguin Random House
15John Le Carre|Berkley
16Alex Michaelides|Simon & Schuster
17Carol Shaben|Simon & Schuster
The statements above perform the following actions:
-
Line 1 starts a
SELECT
statement to get data from the database. -
Line 2 selects the first and last name from the
author
table using thea
alias for theauthor
table and concatenates them together with a space character. -
Line 3 selects the publisher’s name aliased to
publisher_name
. -
Line 4 uses the
author
table as the first source from which to retrieve data and assigns it to the aliasa
. -
Line 5 is the first step of the process outlined above for connecting the
author
table to thepublisher
table. It uses the aliasap
for theauthor_publisher
association table and performs aJOIN
operation to connect theap.author_id
foreign key reference to thea.author_id
primary key in theauthor
table. -
Line 6 is the second step in the two-step process mentioned above. It uses the alias
p
for thepublisher
table and performs aJOIN
operation to relate theap.publisher_id
foreign key reference to thep.publisher_id
primary key in thepublisher
table. -
Line 7 sorts the data by the author’s last name in ascending alphabetical order and ends the SQL query.
-
Lines 8 to 17 are the output of the SQL query.
Note that the data in the source author
and publisher
tables are normalized, with no data duplication. Yet the returned results have duplicated data where necessary to answer the SQL query.
The SQL query above demonstrates how to make use of a relationship using the SQL JOIN
keyword, but the resulting data is a partial re-creation of the author_book_publisher.csv
CSV data. What’s the win for having done the work of creating a database to separate the data?
Here’s another SQL query to show a little bit of the power of SQL and the database engine:
1sqlite> SELECT
2 ...> a.first_name || ' ' || a.last_name AS author_name,
3 ...> COUNT(b.title) AS total_books
4 ...> FROM author a
5 ...> JOIN book b ON b.author_id = a.author_id
6 ...> GROUP BY author_name
7 ...> ORDER BY total_books DESC, a.last_name ASC;
8Stephen King|3
9Tom Clancy|2
10Isaac Asimov|1
11Pearl Buck|1
12John Le Carre|1
13Alex Michaelides|1
14Carol Shaben|1
The SQL query above returns the list of authors and the number of books they’ve written. The list is sorted first by the number of books in descending order, then by the author’s name in alphabetical order:
-
Line 1 begins the SQL query with the
SELECT
keyword. -
Line 2 selects the author’s first and last names, separated by a space character, and creates the alias
author_name
. -
Line 3 counts the number of books written by each author, which will be used later by the
ORDER BY
clause to sort the list. -
Line 4 selects the
author
table to get data from and creates thea
alias. -
Line 5 connects to the related
book
table through aJOIN
to theauthor_id
and creates theb
alias for thebook
table. -
Line 6 generates the aggregated author and total number of books data by using the
GROUP BY
mot-clé.GROUP BY
is what groups eachauthor_name
and controls what books are tallied byCOUNT()
for that author. -
Line 7 sorts the output first by number of books in descending order, then by the author’s last name in ascending alphabetical order.
-
Lines 8 to 14 are the output of the SQL query.
In the above example, you take advantage of SQL to perform aggregation calculations and sort the results into a useful order. Having the database perform calculations based on its built-in data organization ability is usually faster than performing the same kinds of calculations on raw data sets in Python. SQL offers the advantages of using set theory embedded in RDBMS databases.
Entity Relationship Diagrams
An entity-relationship diagram (ERD) is a visual depiction of an entity-relationship model for a database or part of a database. The author_book_publisher.db
SQLite database is small enough that the entire database can be visualized by the diagram shown below:
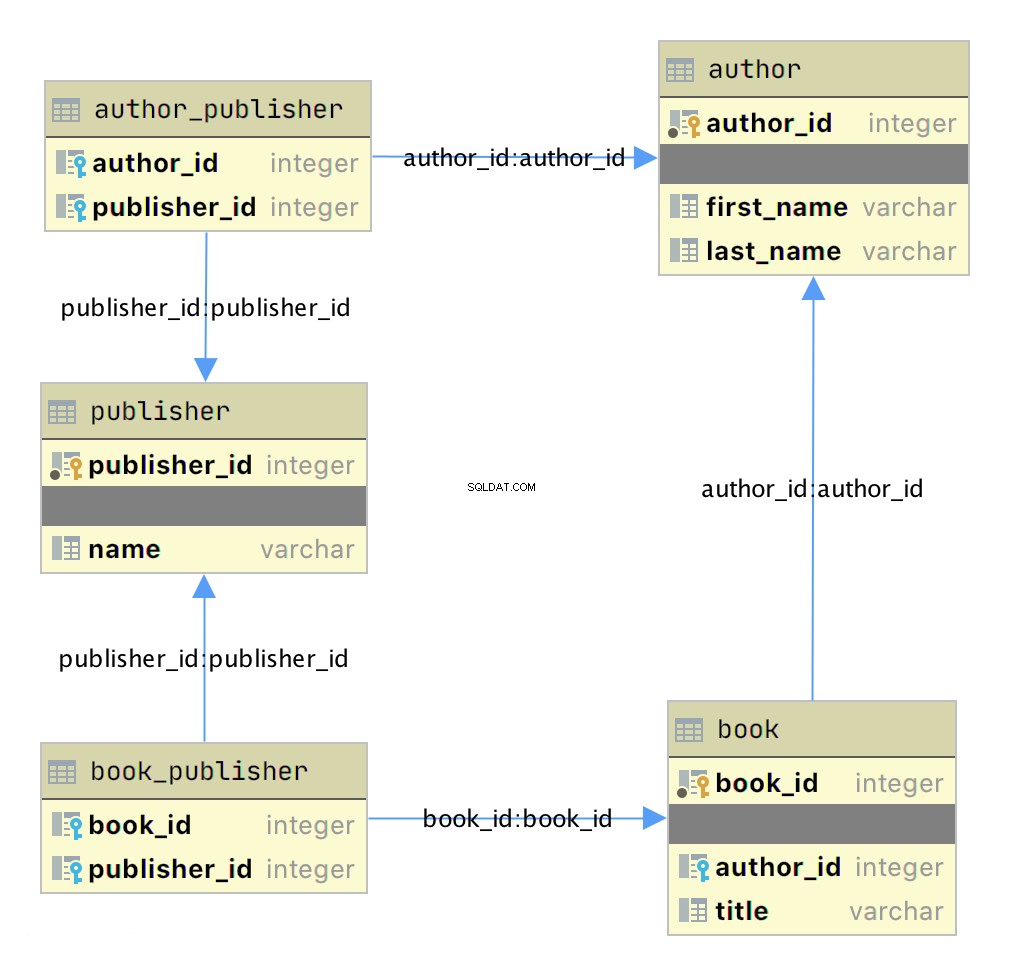
This diagram presents the table structures in the database and the relationships between them. Each box represents a table and contains the fields defined in the table, with the primary key indicated first if it exists.
The arrows show the relationships between the tables connecting a foreign key field in one table to a field, often the primary key, in another table. The table book_publisher
has two arrows, one connecting it to the book
table and another connecting it to the publisher
table. The arrow indicates the many-to-many relationship between the book
and publisher
les tables. The author_publisher
table provides the same relationship between author
and publisher
.
Working With SQLAlchemy and Python Objects
SQLAlchemy is a powerful database access tool kit for Python, with its object-relational mapper (ORM) being one of its most famous components, and the one discussed and used here.
When you’re working in an object-oriented language like Python, it’s often useful to think in terms of objects. It’s possible to map the results returned by SQL queries to objects, but doing so works against the grain of how the database works. Sticking with the scalar results provided by SQL works against the grain of how Python developers work. This problem is known as object-relational impedance mismatch.
The ORM provided by SQLAlchemy sits between the SQLite database and your Python program and transforms the data flow between the database engine and Python objects. SQLAlchemy allows you to think in terms of objects and still retain the powerful features of a database engine.
The Model
One of the fundamental elements to enable connecting SQLAlchemy to a database is creating a model . The model is a Python class defining the data mapping between the Python objects returned as a result of a database query and the underlying database tables.
The entity-relationship diagram displayed earlier shows boxes connected with arrows. The boxes are the tables built with the SQL commands and are what the Python classes will model. The arrows are the relationships between the tables.
The models are Python classes inheriting from an SQLAlchemy Base
classe. The Base
class provides the interface operations between instances of the model and the database table.
Below is the models.py
file that creates the models to represent the author_book_publisher.db
database:
1from sqlalchemy import Column, Integer, String, ForeignKey, Table
2from sqlalchemy.orm import relationship, backref
3from sqlalchemy.ext.declarative import declarative_base
4
5Base = declarative_base()
6
7author_publisher = Table(
8 "author_publisher",
9 Base.metadata,
10 Column("author_id", Integer, ForeignKey("author.author_id")),
11 Column("publisher_id", Integer, ForeignKey("publisher.publisher_id")),
12)
13
14book_publisher = Table(
15 "book_publisher",
16 Base.metadata,
17 Column("book_id", Integer, ForeignKey("book.book_id")),
18 Column("publisher_id", Integer, ForeignKey("publisher.publisher_id")),
19)
20
21class Author(Base):
22 __tablename__ = "author"
23 author_id = Column(Integer, primary_key=True)
24 first_name = Column(String)
25 last_name = Column(String)
26 books = relationship("Book", backref=backref("author"))
27 publishers = relationship(
28 "Publisher", secondary=author_publisher, back_populates="authors"
29 )
30
31class Book(Base):
32 __tablename__ = "book"
33 book_id = Column(Integer, primary_key=True)
34 author_id = Column(Integer, ForeignKey("author.author_id"))
35 title = Column(String)
36 publishers = relationship(
37 "Publisher", secondary=book_publisher, back_populates="books"
38 )
39
40class Publisher(Base):
41 __tablename__ = "publisher"
42 publisher_id = Column(Integer, primary_key=True)
43 name = Column(String)
44 authors = relationship(
45 "Author", secondary=author_publisher, back_populates="publishers"
46 )
47 books = relationship(
48 "Book", secondary=book_publisher, back_populates="publishers"
49 )
Here’s what’s going on in this module:
-
Line 1 imports the
Column
,Integer
,String
,ForeignKey
, andTable
classes from SQLAlchemy, which are used to help define the model attributes. -
Line 2 imports the
relationship()
andbackref
objects, which are used to create the relationships between objects. -
Line 3 imports the
declarative_base
object, which connects the database engine to the SQLAlchemy functionality of the models. -
Line 5 creates the
Base
class, which is what all models inherit from and how they get SQLAlchemy ORM functionality. -
Lines 7 to 12 create the
author_publisher
association table model. -
Lines 14 to 19 create the
book_publisher
association table model. -
Lines 21 to 29 define the
Author
class model to theauthor
database table. -
Lines 31 to 38 define the
Book
class model to thebook
database table. -
Lines 40 to 49 define the
Publisher
class model to thepublisher
database table.
The description above shows the mapping of the five tables in the author_book_publisher.db
base de données. But it glosses over some SQLAlchemy ORM features, including Table
, ForeignKey
, relationship()
, and backref
. Let’s get into those now.
Table
Creates Associations
author_publisher
and book_publisher
are both instances of the Table
class that create the many-to-many association tables used between the author
and publisher
tables and the book
and publisher
tables, respectively.
The SQLAlchemy Table
class creates a unique instance of an ORM mapped table within the database. The first parameter is the table name as defined in the database, and the second is Base.metadata
, which provides the connection between the SQLAlchemy functionality and the database engine.
The rest of the parameters are instances of the Column
class defining the table fields by name, their type, and in the example above, an instance of a ForeignKey
.
ForeignKey
Creates a Connection
The SQLAlchemy ForeignKey
class defines a dependency between two Column
fields in different tables. A ForeignKey
is how you make SQLAlchemy aware of the relationships between tables. For example, this line from the author_publisher
instance creation establishes a foreign key relationship:
Column("author_id", Integer, ForeignKey("author.author_id"))
The statement above tells SQLAlchemy that there’s a column in the author_publisher
table named author_id
. The type of that column is Integer
, and author_id
is a foreign key related to the primary key in the author
table.
Having both author_id
and publisher_id
defined in the author_publisher
Table
instance creates the connection from the author
table to the publisher
table and vice versa, establishing a many-to-many relationship.
relationship()
Establishes a Collection
Having a ForeignKey
defines the existence of the relationship between tables but not the collection of books an author can have. Take a look at this line in the Author
class definition:
books = relationship("Book", backref=backref("author"))
The code above defines a parent-child collection. The books
attribute being plural (which is not a requirement, just a convention) is an indication that it’s a collection.
The first parameter to relationship()
, the class name Book
(which is not the table name book
), is the class to which the books
attribute is related. The relationship
informs SQLAlchemy that there’s a relationship between the Author
and Book
classes. SQLAlchemy will find the relationship in the Book
class definition:
author_id = Column(Integer, ForeignKey("author.author_id"))
SQLAlchemy recognizes that this is the ForeignKey
connection point between the two classes. You’ll get to the backref
parameter in relationship()
in a moment.
The other relationship in Author
is to the Publisher
classe. This is created with the following statement in the Author
class definition:
publishers = relationship(
"Publisher", secondary=author_publisher, back_populates="authors"
)
Like books
, the attribute publishers
indicates a collection of publishers associated with an author. The first parameter, "Publisher"
, informs SQLAlchemy what the related class is. The second and third parameters are secondary=author_publisher
and back_populates="authors"
:
-
secondary
tells SQLAlchemy that the relationship to thePublisher
class is through a secondary table, which is theauthor_publisher
association table created earlier inmodels.py
. Thesecondary
parameter makes SQLAlchemy find thepublisher_id
ForeignKey
defined in theauthor_publisher
association table. -
back_populates
is a convenience configuration telling SQLAlchemy that there’s a complementary collection in thePublisher
class calledauthors
.
backref
Mirrors Attributes
The backref
parameter of the books
collection relationship()
creates an author
attribute for each Book
instance. This attribute refers to the parent Author
that the Book
instance is related to.
For example, if you executed the following Python code, then a Book
instance would be returned from the SQLAlchemy query. The Book
instance has attributes that can be used to print out information about the book:
book = session.query(Book).filter_by(Book.title == "The Stand").one_or_none()
print(f"Authors name: {book.author.first_name} {book.author.last_name}")
The existence of the author
attribute in the Book
above is because of the backref
définition. A backref
can be very handy to have when you need to refer to the parent and all you have is a child instance.
Queries Answer Questions
You can make a basic query like SELECT * FROM author;
in SQLAlchemy like this:
results = session.query(Author).all()
The session
is an SQLAlchemy object used to communicate with SQLite in the Python example programs. Here, you tell the session you want to execute a query against the Author
model and return all records.
At this point, the advantages of using SQLAlchemy instead of plain SQL might not be obvious, especially considering the setup required to create the models representing the database. The results
returned by the query is where the magic happens. Instead of getting back a list of lists of scalar data, you’ll get back a list of instances of Author
objects with attributes matching the column names you defined.
The books
and publishers
collections maintained by SQLAlchemy create a hierarchical list of authors and the books they’ve written as well as the publishers who’ve published them.
Behind the scenes, SQLAlchemy turns the object and method calls into SQL statements to execute against the SQLite database engine. SQLAlchemy transforms the data returned by SQL queries into Python objects.
With SQLAlchemy, you can perform the more complex aggregation query shown earlier for the list of authors and the number of books they’ve written like this:
author_book_totals = (
session.query(
Author.first_name,
Author.last_name,
func.count(Book.title).label("book_total")
)
.join(Book)
.group_by(Author.last_name)
.order_by(desc("book_total"))
.all()
)
The query above gets the author’s first and last name, along with a count of the number of books that the author has written. The aggregating count
used by the group_by
clause is based on the author’s last name. Finally, the results are sorted in descending order based on the aggregated and aliased book_total
.
Example Program
The example program examples/example_2/main.py
has the same functionality as examples/example_1/main.py
but uses SQLAlchemy exclusively to interface with the author_book_publisher.db
SQLite database. The program is broken up into the main()
function and the functions it calls:
1def main():
2 """Main entry point of program"""
3 # Connect to the database using SQLAlchemy
4 with resources.path(
5 "project.data", "author_book_publisher.db"
6 ) as sqlite_filepath:
7 engine = create_engine(f"sqlite:///{sqlite_filepath}")
8 Session = sessionmaker()
9 Session.configure(bind=engine)
10 session = Session()
11
12 # Get the number of books printed by each publisher
13 books_by_publisher = get_books_by_publishers(session, ascending=False)
14 for row in books_by_publisher:
15 print(f"Publisher: {row.name}, total books: {row.total_books}")
16 print()
17
18 # Get the number of authors each publisher publishes
19 authors_by_publisher = get_authors_by_publishers(session)
20 for row in authors_by_publisher:
21 print(f"Publisher: {row.name}, total authors: {row.total_authors}")
22 print()
23
24 # Output hierarchical author data
25 authors = get_authors(session)
26 output_author_hierarchy(authors)
27
28 # Add a new book
29 add_new_book(
30 session,
31 author_name="Stephen King",
32 book_title="The Stand",
33 publisher_name="Random House",
34 )
35 # Output the updated hierarchical author data
36 authors = get_authors(session)
37 output_author_hierarchy(authors)
This program is a modified version of examples/example_1/main.py
. Let’s go over the differences:
-
Lines 4 to 7 first initialize the
sqlite_filepath
variable to the database file path. Then they create theengine
variable to communicate with SQLite and theauthor_book_publisher.db
database file, which is SQLAlchemy’s access point to the database. -
Line 8 creates the
Session
class from the SQLAlchemy’ssessionmaker()
. -
Line 9 binds the
Session
to the engine created in line 8. -
Line 10 creates the
session
instance, which is used by the program to communicate with SQLAlchemy.
The rest of the function is similar, except for the replacement of data
with session
as the first parameter to all the functions called by main()
.
get_books_by_publisher()
has been refactored to use SQLAlchemy and the models you defined earlier to get the data requested:
1def get_books_by_publishers(session, ascending=True):
2 """Get a list of publishers and the number of books they've published"""
3 if not isinstance(ascending, bool):
4 raise ValueError(f"Sorting value invalid: {ascending}")
5
6 direction = asc if ascending else desc
7
8 return (
9 session.query(
10 Publisher.name, func.count(Book.title).label("total_books")
11 )
12 .join(Publisher.books)
13 .group_by(Publisher.name)
14 .order_by(direction("total_books"))
15 )
Here’s what the new function, get_books_by_publishers()
, is doing:
-
Line 6 creates the
direction
variable and sets it equal to the SQLAlchemydesc
orasc
function depending on the value of theascending
paramètre. -
Lines 9 to 11 query the
Publisher
table for data to return, which in this case arePublisher.name
and the aggregate total ofBook
objects associated with an author, aliased tototal_books
. -
Line 12 joins to the
Publisher.books
collecte. -
Line 13 aggregates the book counts by the
Publisher.name
attribute. -
Line 14 sorts the output by the book counts according to the operator defined by
direction
. -
Line 15 closes the object, executes the query, and returns the results to the caller.
All the above code expresses what is wanted rather than how it’s to be retrieved. Now instead of using SQL to describe what’s wanted, you’re using Python objects and methods. What’s returned is a list of Python objects instead of a list of tuples of data.
get_authors_by_publisher()
has also been modified to work exclusively with SQLAlchemy. Its functionality is very similar to the previous function, so a function description is omitted:
def get_authors_by_publishers(session, ascending=True):
"""Get a list of publishers and the number of authors they've published"""
if not isinstance(ascending, bool):
raise ValueError(f"Sorting value invalid: {ascending}")
direction = asc if ascending else desc
return (
session.query(
Publisher.name,
func.count(Author.first_name).label("total_authors"),
)
.join(Publisher.authors)
.group_by(Publisher.name)
.order_by(direction("total_authors"))
)
get_authors()
has been added to get a list of authors sorted by their last names. The result of this query is a list of Author
objects containing a collection of books. The Author
objects already contain hierarchical data, so the results don’t have to be reformatted:
def get_authors(session):
"""Get a list of author objects sorted by last name"""
return session.query(Author).order_by(Author.last_name).all()
Like its previous version, add_new_book()
is relatively complex but straightforward to understand. It determines if a book with the same title, author, and publisher exists in the database already.
If the search query finds an exact match, then the function returns. If no book matches the exact search criteria, then it searches to see if the author has written a book using the passed in title. This code exists to prevent duplicate books from being created in the database.
If no matching book exists, and the author hasn’t written one with the same title, then a new book is created. The function then retrieves or creates an author and publisher. Once instances of the Book
, Author
and Publisher
exist, the relationships between them are created, and the resulting information is saved to the database:
1def add_new_book(session, author_name, book_title, publisher_name):
2 """Adds a new book to the system"""
3 # Get the author's first and last names
4 first_name, _, last_name = author_name.partition(" ")
5
6 # Check if book exists
7 book = (
8 session.query(Book)
9 .join(Author)
10 .filter(Book.title == book_title)
11 .filter(
12 and_(
13 Author.first_name == first_name, Author.last_name == last_name
14 )
15 )
16 .filter(Book.publishers.any(Publisher.name == publisher_name))
17 .one_or_none()
18 )
19 # Does the book by the author and publisher already exist?
20 if book is not None:
21 return
22
23 # Get the book by the author
24 book = (
25 session.query(Book)
26 .join(Author)
27 .filter(Book.title == book_title)
28 .filter(
29 and_(
30 Author.first_name == first_name, Author.last_name == last_name
31 )
32 )
33 .one_or_none()
34 )
35 # Create the new book if needed
36 if book is None:
37 book = Book(title=book_title)
38
39 # Get the author
40 author = (
41 session.query(Author)
42 .filter(
43 and_(
44 Author.first_name == first_name, Author.last_name == last_name
45 )
46 )
47 .one_or_none()
48 )
49 # Do we need to create the author?
50 if author is None:
51 author = Author(first_name=first_name, last_name=last_name)
52 session.add(author)
53
54 # Get the publisher
55 publisher = (
56 session.query(Publisher)
57 .filter(Publisher.name == publisher_name)
58 .one_or_none()
59 )
60 # Do we need to create the publisher?
61 if publisher is None:
62 publisher = Publisher(name=publisher_name)
63 session.add(publisher)
64
65 # Initialize the book relationships
66 book.author = author
67 book.publishers.append(publisher)
68 session.add(book)
69
70 # Commit to the database
71 session.commit()
The code above is relatively long. Let’s break the functionality down to manageable sections:
-
Lines 7 to 18 set the
book
variable to an instance of aBook
if a book with the same title, author, and publisher is found. Otherwise, they setbook
toNone
. -
Lines 20 and 21 determine if the book already exists and return if it does.
-
Lines 24 to 37 set the
book
variable to an instance of aBook
if a book with the same title and author is found. Otherwise, they create a newBook
instance. -
Lines 40 to 52 set the
author
variable to an existing author, if found, or create a newAuthor
instance based on the passed-in author name. -
Lines 55 to 63 set the
publisher
variable to an existing publisher, if found, or create a newPublisher
instance based on the passed-in publisher name. -
Line 66 sets the
book.author
instance to theauthor
instance. This creates the relationship between the author and the book, which SQLAlchemy will create in the database when the session is committed. -
Line 67 adds the
publisher
instance to thebook.publishers
collection. This creates the many-to-many relationship between thebook
andpublisher
les tables. SQLAlchemy will create references in the tables as well as in thebook_publisher
association table that connects the two. -
Line 68 adds the
Book
instance to the session, making it part of the session’s unit of work. -
Line 71 commits all the creations and updates to the database.
There are a few things to take note of here. First, there’s is no mention of the author_publisher
or book_publisher
association tables in either the queries or the creations and updates. Because of the work you did in models.py
setting up the relationships, SQLAlchemy can handle connecting objects together and keeping those tables in sync during creations and updates.
Second, all the creations and updates happen within the context of the session
objet. None of that activity is touching the database. Only when the session.commit()
statement executes does the session then go through its unit of work and commit that work to the database.
For example, if a new Book
instance is created (as in line 37 above), then the book has its attributes initialized except for the book_id
primary key and author_id
foreign key. Because no database activity has happened yet, the book_id
is unknown, and nothing was done in the instantiation of book
to give it an author_id
.
When session.commit()
is executed, one of the things it will do is insert book
into the database, at which point the database will create the book_id
primary key. The session will then initialize the book.book_id
value with the primary key value created by the database engine.
session.commit()
is also aware of the insertion of the Book
instance in the author.books
collection. The author
object’s author_id
primary key will be added to the Book
instance appended to the author.books
collection as the author_id
foreign key.
Providing Access to Multiple Users
To this point, you’ve seen how to use pandas, SQLite, and SQLAlchemy to access the same data in different ways. For the relatively straightforward use case of the author, book, and publisher data, it could still be a toss-up whether you should use a database.
One deciding factor when choosing between using a flat file or a database is data and relationship complexity. If the data for each entity is complicated and contains many relationships between the entities, then creating and maintaining it in a flat file might become more difficult.
Another factor to consider is whether you want to share the data between multiple users. The solution to this problem might be as simple as using a sneakernet to physically move data between users. Moving data files around this way has the advantage of ease of use, but the data can quickly get out of sync when changes are made.
The problem of keeping the data consistent for all users becomes even more difficult if the users are remote and want to access the data across networks. Even when you’re limited to a single language like Python and using pandas to access the data, network file locking isn’t sufficient to ensure the data doesn’t get corrupted.
Providing the data through a server application and a user interface alleviates this problem. The server is the only application that needs file-level access to the database. By using a database, the server can take advantage of SQL to access the data using a consistent interface no matter what programming language the server uses.
The last example program demonstrates this by providing a web application and user interface to the Chinook sample SQLite database. Peter Stark generously maintains the Chinook database as part of the SQLite Tutorial site. If you’d like to learn more about SQLite and SQL in general, then the site is a great resource.
The Chinook database provides artist, music, and playlist information along the lines of a simplified Spotify. The database is part of the example code project in the project/data
folder.
Using Flask With Python, SQLite, and SQLAlchemy
The examples/example_3/chinook_server.py
program creates a Flask application that you can interact with using a browser. The application makes use of the following technologies:
-
Flask Blueprint is part of Flask and provides a good way to follow the separation of concerns design principle and create distinct modules to contain functionality.
-
Flask SQLAlchemy is an extension for Flask that adds support for SQLAlchemy in your web applications.
-
Flask_Bootstrap4 packages the Bootstrap front-end tool kit, integrating it with your Flask web applications.
-
Flask_WTF extends Flask with WTForms, giving your web applications a useful way to generate and validate web forms.
-
python_dotenv is a Python module that an application uses to read environment variables from a file and keep sensitive information out of program code.
Though not necessary for this example, a .env
file holds the environment variables for the application. The .env
file exists to contain sensitive information like passwords, which you should keep out of your code files. However, the content of the project .env
file is shown below since it doesn’t contain any sensitive data:
SECRET_KEY = "you-will-never-guess"
SQLALCHEMY_TRACK_MODIFICATIONS = False
SQLAlCHEMY_ECHO = False
DEBUG = True
The example application is fairly large, and only some of it is relevant to this tutorial. For this reason, examining and learning from the code is left as an exercise for the reader. That said, you can take a look at an animated screen capture of the application below, followed by the HTML that renders the home page and the Python Flask route that provides the dynamic data.
Here’s the application in action, navigating through various menus and features:
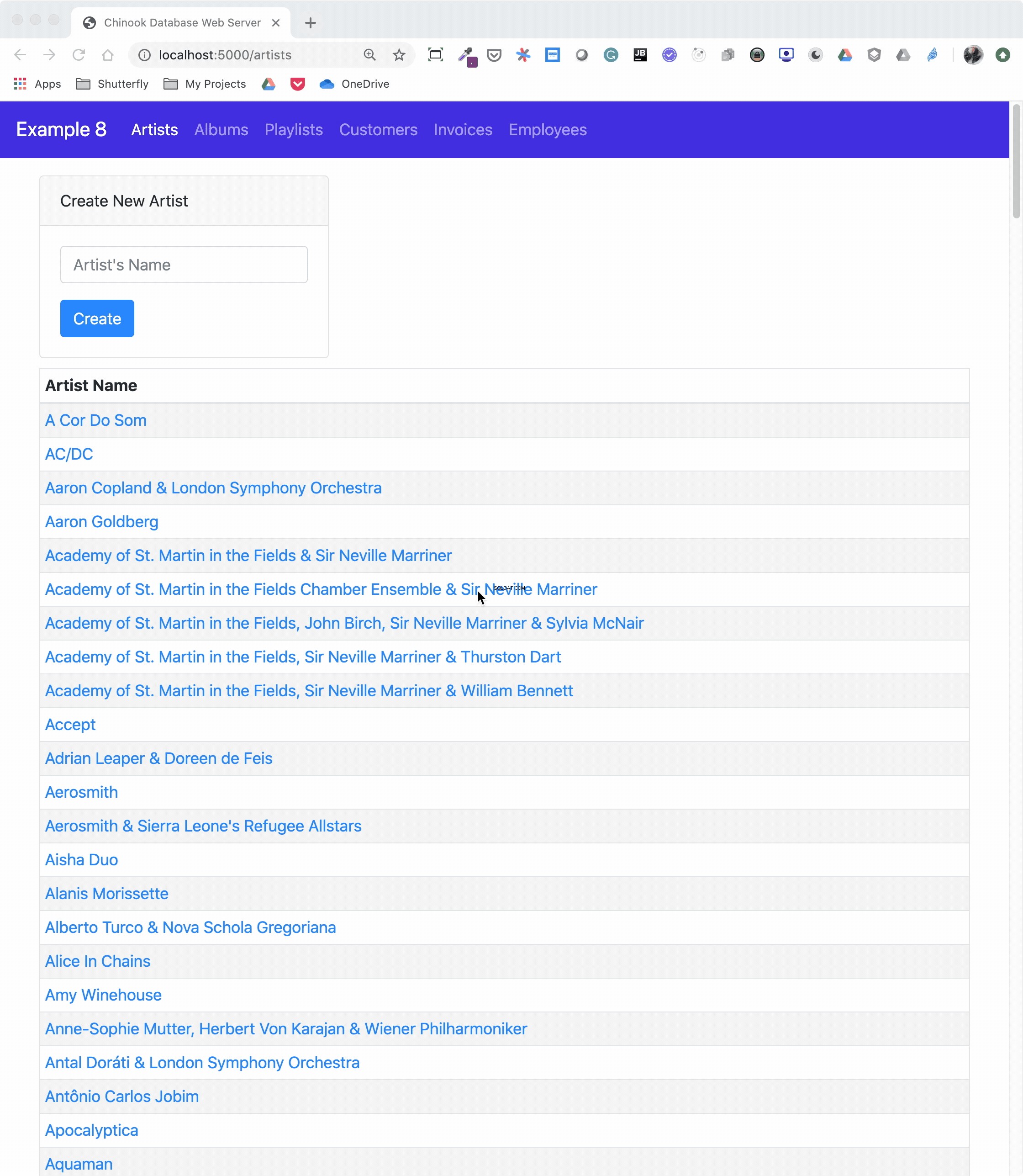
The animated screen capture starts on the application home page, styled using Bootstrap 4. The page displays the artists in the database, sorted in ascending order. The remainder of the screen capture presents the results of clicking on the displayed links or navigating around the application from the top-level menu.
Here’s the Jinja2 HTML template that generates the home page of the application:
1{% extends "base.html" %}
2
3{% block content %}
4<div class="container-fluid">
5 <div class="m-4">
6 <div class="card" style="width: 18rem;">
7 <div class="card-header">Create New Artist</div>
8 <div class="card-body">
9 <form method="POST" action="{{url_for('artists_bp.artists')}}">
10 {{ form.csrf_token }}
11 {{ render_field(form.name, placeholder=form.name.label.text) }}
12 <button type="submit" class="btn btn-primary">Create</button>
13 </form>
14 </div>
15 </div>
16 <table class="table table-striped table-bordered table-hover table-sm">
17 <caption>List of Artists</caption>
18 <thead>
19 <tr>
20 <th>Artist Name</th>
21 </tr>
22 </thead>
23 <tbody>
24 {% for artist in artists %}
25 <tr>
26 <td>
27 <a href="{{url_for('albums_bp.albums', artist_id=artist.artist_id)}}">
28 {{ artist.name }}
29 </a>
30 </td>
31 </tr>
32 {% endfor %}
33 </tbody>
34 </table>
35 </div>
36</div>
37{% endblock %}
Here’s what’s going on in this Jinja2 template code:
-
Line 1 uses Jinja2 template inheritance to build this template from the
base.html
modèle. Thebase.html
template contains all the HTML5 boilerplate code as well as the Bootstrap navigation bar consistent across all pages of the site. -
Lines 3 to 37 contain the block content of the page, which is incorporated into the Jinja2 macro of the same name in the
base.html
base template. -
Lines 9 to 13 render the form to create a new artist. This uses the features of Flask-WTF to generate the form.
-
Lines 24 to 32 create a
for
loop that renders the table of artist names. -
Lines 27 to 29 render the artist name as a link to the artist’s album page showing the songs associated with a particular artist.
Here’s the Python route that renders the page:
1from flask import Blueprint, render_template, redirect, url_for
2from flask_wtf import FlaskForm
3from wtforms import StringField
4from wtforms.validators import InputRequired, ValidationError
5from app import db
6from app.models import Artist
7
8# Set up the blueprint
9artists_bp = Blueprint(
10 "artists_bp", __name__, template_folder="templates", static_folder="static"
11)
12
13def does_artist_exist(form, field):
14 artist = (
15 db.session.query(Artist)
16 .filter(Artist.name == field.data)
17 .one_or_none()
18 )
19 if artist is not None:
20 raise ValidationError("Artist already exists", field.data)
21
22class CreateArtistForm(FlaskForm):
23 name = StringField(
24 label="Artist's Name", validators=[InputRequired(), does_artist_exist]
25 )
26
27@artists_bp.route("/")
28@artists_bp.route("/artists", methods=["GET", "POST"])
29def artists():
30 form = CreateArtistForm()
31
32 # Is the form valid?
33 if form.validate_on_submit():
34 # Create new artist
35 artist = Artist(name=form.name.data)
36 db.session.add(artist)
37 db.session.commit()
38 return redirect(url_for("artists_bp.artists"))
39
40 artists = db.session.query(Artist).order_by(Artist.name).all()
41 return render_template("artists.html", artists=artists, form=form,)
Let’s go over what the above code is doing:
-
Lines 1 to 6 import all the modules necessary to render the page and initialize forms with data from the database.
-
Lines 9 to 11 create the blueprint for the artists page.
-
Lines 13 to 20 create a custom validator function for the Flask-WTF forms to make sure a request to create a new artist doesn’t conflict with an already existing artist.
-
Lines 22 to 25 create the form class to handle the artist form rendered in the browser and provide validation of the form field inputs.
-
Lines 27 to 28 connect two routes to the
artists()
function they decorate. -
Line 30 creates an instance of the
CreateArtistForm()
class. -
Line 33 determines if the page was requested through the HTTP methods GET or POST (submit). If it was a POST, then it also validates the fields of the form and informs the user if the fields are invalid.
-
Lines 35 to 37 create a new artist object, add it to the SQLAlchemy session, and commit the artist object to the database, persisting it.
-
Line 38 redirects back to the artists page, which will be rerendered with the newly created artist.
-
Line 40 runs an SQLAlchemy query to get all the artists in the database and sort them by
Artist.name
. -
Line 41 renders the artists page if the HTTP request method was a GET.
You can see that a great deal of functionality is created by a reasonably small amount of code.
Creating a REST API Server
You can also create a web server providing a REST API. This kind of server offers URL endpoints responding with data, often in JSON format. A server providing REST API endpoints can be used by JavaScript single-page web applications through the use of AJAX HTTP requests.
Flask is an excellent tool for creating REST applications. For a multi-part series of tutorials about using Flask, Connexion, and SQLAlchemy to create REST applications, check out Python REST APIs With Flask, Connexion, and SQLAlchemy.
If you’re a fan of Django and are interested in creating REST APIs, then check out Django Rest Framework – An Introduction and Create a Super Basic REST API with Django Tastypie.
Note: It’s reasonable to ask if SQLite is the right choice as the database backend to a web application. The SQLite website states that SQLite is a good choice for sites that serve around 100,000 hits per day. If your site gets more daily hits, the first thing to say is congratulations!
Beyond that, if you’ve implemented your website with SQLAlchemy, then it’s possible to move the data from SQLite to another database such as MySQL or PostgreSQL. For a comparison of SQLite, MySQL, and PostgreSQL that will help you make decisions about which one will serve your application best, check out Introduction to Python SQL Libraries.
It’s well worth considering SQLite for your Python application, no matter what it is. Using a database gives your application versatility, and it might create surprising opportunities to add additional features.
Conclusion
You’ve covered a lot of ground in this tutorial about databases, SQLite, SQL, and SQLAlchemy! You’ve used these tools to move data contained in flat files to an SQLite database, access the data with SQL and SQLAlchemy, and provide that data through a web server.
In this tutorial, you’ve learned:
- Why an SQLite database can be a compelling alternative to flat-file data storage
- How to normalize data to reduce data redundancy and increase data integrity
- How to use SQLAlchemy to work with databases in an object-oriented manner
- How to build a web application to serve a database to multiple users
Working with databases is a powerful abstraction for working with data that adds significant functionality to your Python programs and allows you to ask interesting questions of your data.
You can get all of the code and data you saw in this tutorial at the link below:
Download the sample code: Click here to get the code you’ll use to learn about data management with SQLite and SQLAlchemy in this tutorial.
Further Reading
This tutorial is an introduction to using databases, SQL, and SQLAlchemy, but there’s much more to learn about these subjects. These are powerful, sophisticated tools that no single tutorial can cover adequately. Here are some resources for additional information to expand your skills:
-
If your application will expose the database to users, then avoiding SQL injection attacks is an important skill. For more information, check out Preventing SQL Injection Attacks With Python.
-
Providing web access to a database is common in web-based single-page applications. To learn how, check out Python REST APIs With Flask, Connexion, and SQLAlchemy – Part 2.
-
Preparing for data engineering job interviews gives you a leg up in your career. To get started, check out Data Engineer Interview Questions With Python.
-
Migrating data and being able to roll back using Flask with Postgres and SQLAlchemy is an integral part of the Software Development Life Cycle (SDLC). You can learn more about it by checking out Flask by Example – Setting up Postgres, SQLAlchemy, and Alembic.